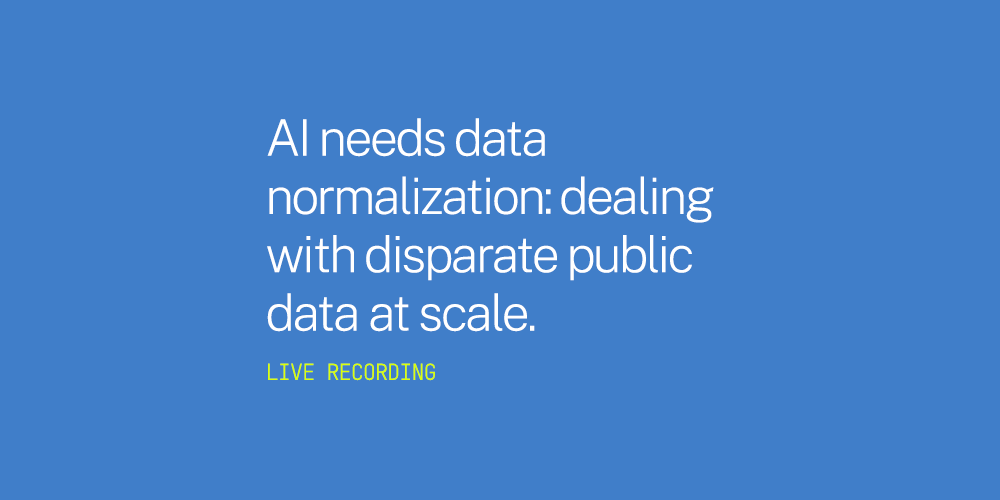
AI needs data normalization: dealing with disparate public data
September 27, 2021
Discover the Latest in Land Parcels & Spatial Data with the Regrid Team!
This past week on September 23rd, 2021 Regrid sponsored and co-hosted a virtual event 'The challenges of working with public data at scale' with Geoawesomeness. Working with Muthu and Aleks was a pleasure and we cannot wait to continue our partnership in the future. Looking forward to more amazing webinars about parcel data, property, and more.
Here are all four presentations from the event, separated by topic.
1. Matt Hampel (Regrid's Chief Data Officer) - 'Falsehoods Programs Believe About Parcels'
2. Nancy von Meyer (President, Fairview Industries) - 'The Parcel Challenge: Making the Complex Simple'
3. Pablo Fuentes & Brendan Collins (Founder and Principal at makepath) - 'Normalizing Public Data at Scale'
4. Scott Simmons (Open Geospatial Consortium) - 'Ensuring confidence in land administration information'
Thank you to everyone who attended the event and for all of the positive feedback - we look forward to hosting more events like this in the future. Make sure to follow Regrid and Geoawesomeness on your social platforms to stay updated with the latest news.
More about the event:
We have all heard and read about the difficulties public organizations and health agencies have had sharing spatial data with each other due to a myriad of issues. Working with public datasets at scale is a challenge. For many of us, it involves finding, cleaning, and standardizing disparate local datasets from over 3000 counties just in the USA. Now imagine the complexities across national boundaries and with the data structure in each county. Every county and state does its data differently, and there is no one standard for normalizing this data... And it's a deeply human problem -- AI just won't cut it. We've put together a stellar panel for this event as we discuss the importance of standardization when working with disparate public datasets.